The journey begins as Kate Keahey’s wandering path unfolds, leading to improbable events. Keahey, Senior Scientist at Argonne National Laboratory and the University of Chicago, leads Chameleon. This innovative project offers an edge-to-cloud platform tailored for experimental computer science research, educational endeavors, and emerging applications. Chameleon essentially functions as a bare-metal, reconfigurable testbed, facilitating researchers in exploring various system-related subjects such as energy efficiency in computing, performance variability, and experimentation with unconventional protocols, algorithms, or performance trade-offs. Its scope spans a broad spectrum, encompassing topics from systems research to machine learning and artificial intelligence.
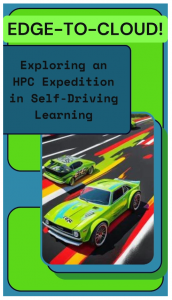
Several years ago, the Chameleon team observed a growing interest in edge computing, with the key goal of bringing computational capabilities closer to the edge where data originates. This approach often involves employing lightweight devices such as Raspberry Pis or NVIDIA Nanos outside traditional data centers, forming what is known as edge-to-cloud infrastructure. This strategy aims to process data at its source, minimizing latency, reducing network congestion, and enhancing system responsiveness. Chameleon facilitates edge-to-cloud experimentation from a single Jupyter Notebook and simultaneously enables creating and sharing documents containing live code, equations, visualizations, and explanatory text.
These experiments typically involve combining real-time data acquisition and processing at the edge with the utilization of specialized hardware, including GPU resources, which employ AI to analyze data for valuable insights or to train and refine models. These models can then be deployed at the edge to provide immediate feedback. To support such experiments, Chameleon developed a new capability, CHI@Edge, enabling users to integrate their edge resources to the testbed and program complex experimental edge-to-cloud workflows from one Jupyter Notebook.
To discuss the requirements and dynamics of such experiments, a few years back, the group organized the Chameleon User Meeting that focused on edge-to-cloud experimentation. This is where Keahey met Rick Anderson. Anderson, from Rutgers University, caught the team’s attention because he utilized CHI@Edge alongside an open-source DIY self-driving platform to teach classes using self-driving cars.
This meeting was tailored to Keahey’s goal of bringing open-source educational platforms within CHI@Edge. As a self-driving platform for small-scale experimentation, Donkeycar was one of the best options in terms of using an innovative platform for self-driving hobby remote-control cars, catering to enthusiasts and developers in the field of machine learning (ML), autonomous vehicles (AVs), and featuring the use of Raspberry Pis within Supervised Learning locally on a laptop but also utilizing the cloud.
Donkeycar also supports three main autopilot modes: deep learning, path following, and computer vision. The deep learning autopilot utilizes convolutional neural networks (CNNs) to replicate human driving behaviors through behavioral cloning, utilizing camera images, throttle, and steering values for training. The path-following autopilot mode, which utilizes GPS data for steering adjustments relative to a predefined path, is suitable for outdoor environments. The computer vision autopilot employs traditional computer vision techniques to extract features from camera images for steering and throttle commands, offering flexibility in algorithm selection and parameter tuning.
This combined approach offers a fun pathway to introduce learners to AI and machine learning.
“But capturing experimentation as just code is not enough,” says Keahey, “because if you don’t have the resources on which the code can run, you can’t do much. This is particularly true of AI where making progress relies on having access to powerful GPU resources. And it is especially true when constructing an AI-based edge-to-cloud platform.”
With the integration of Donkeycar with CHI@Edge, students can experience the entire cycle, starting from data acquisition, progressing through training, and concluding with validation. This immersive process allows them to observe their endeavors’ outcomes in an engaging learning environment, and Chameleon testbed resources resolve this difficulty.
Keahey highlights the essential components for any research or educational endeavor involving self-driving cars, emphasizing the need for acquiring the vehicles, setting up a suitable testing lab, accessing GPUs for model training, and possessing the necessary expertise to design a comprehensive curriculum. She points out that the feasibility of purchasing small-scale cars integrated with edge devices has opened up new possibilities. Consequently, Chameleon purchased several kits from Waveshare, which are equipped with standard RC Car chassis, steering servo, drive motor, and an adapter board featuring motor controllers, battery charger, battery management circuitry, as well as provisions for connecting a Raspberry Pi and camera. The kits also include a track, tape, custom-track cones, and a wireless gamepad for manual driving, facilitating data collection. The primary goal was to investigate integrating the Donkeycar model into its platform, aiming to blend the self-driving framework with edge-to-cloud resources, ultimately providing students with a practical and accessible solution.
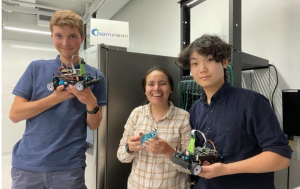
Keahey, also a mentor in the BigDataX REU site, funded by the National Science Foundation (NSF), recruited two students through the program during the summer of 2023: Kyle Zheng, a fourth-year student at Modesto Junior College, and William Fowler from Tufts University. They were REUs (Research Experience for Undergraduates). In addition, Alicia Esquivel Morel, a graduate student from the University of Missouri, was brought on board to share her expertise in edge-related research as a mentor.
Upon completing the module, Zheng and Fowler proceeded to independently develop their own research projects. Keahey shares, “This was an unexpected outcome highlighting the framework’s dual purpose: not only did it serve as a platform for learning, but it also provided a solid foundation for starting their respective independent research projects.”
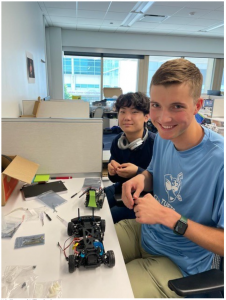
Their research resulted in a paper, “AutoLearn: Learning in the Edge to Cloud Continuum,” co-authored by Michael Sherman, Senior Software Engineer at the University of Chicago, Esquivel, Anderson, and Keahey.
The paper explores a comprehensive examination of the trade-offs between edge and HPC cloud inferencing within the realm of autonomous vehicles.
The study addressed the inherent resource constraints associated with edge inference in self-driving cars by introducing an innovative cloud-aided real-time inferencing framework. Leveraging cloud resources to complement edge devices, this framework offered a modular solution to potential bottlenecks in vehicle actuation.
Inspired by a paper from NVIDIA, the study adopted a conceptual reproduction methodology to assess the feasibility and effectiveness of the proposed framework. Central to the investigation was comparing pure-edge and cloud-aided models regarding resource utilization and autonomy scores.
Experimental findings also revealed that cloud-aided models outperformed edge models, particularly LSTM (Long Short-Term Memory) models, which demanded more computational resources due to their recurrent nature. The study highlighted the limitations faced by LSTM models on the edge, resulting in under-performance and diminished autonomy scores. However, when HPC inferencing was offloaded to the cloud, LSTM models exhibited notable improvements in autonomy, underscoring the potential value of RNNs/LSTMs in self-driving applications.
The paper also explored various factors impacting model performance, including hardware scalability (RPi4 vs. NVIDIA DRIVE), architectural modifications in neural networks, and the volume of training data. Notably, the study observed that cloud-aided models demonstrated enhanced autonomy and efficiency, particularly in tasks such as obstacle detection and navigation.
Integrating cloud computing in the framework proposed in this paper presents a promising avenue for enhancing the autonomy and efficiency of self-driving vehicles. By offloading computational tasks to the cloud, the framework addresses resource constraints inherent in edge devices, thereby laying the groundwork for future advancements in autonomous vehicle technology. This study provides valuable insights into the trade-offs associated with edge and cloud inferencing in self-driving vehicles, highlighting the potential of cloud-aided frameworks to overcome the limitations of edge devices and drive advancements in autonomous vehicle technology into new realms.
Keahey’s dedication to mentoring and guiding students in their exploration of cutting-edge technologies reflects her commitment to nurturing the next generation of innovators and problem solvers. Through her leadership and expertise, she continues to shape the future of AI and autonomous systems, inspiring students to pursue their passions and make meaningful contributions to the world.